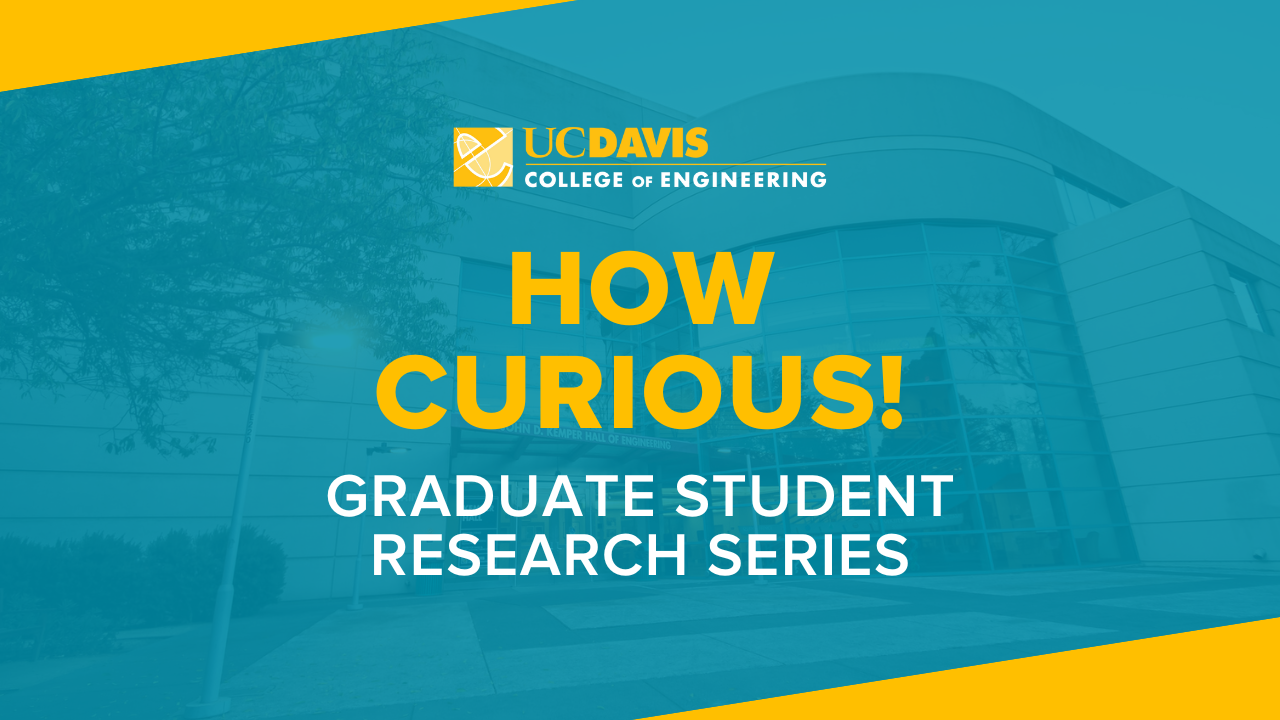
Event Date
How Curious! offers graduate students in master's and Ph.D. programs in the College of Engineering an opportunity to present their research findings and engage in scholarly discussions with their peers and faculty members. The goal is to foster collaboration, encourage intellectual exchange and support the professional development of our graduate student community.
Presenters
Fox Thorpe, Chemical Engineering
Presenting: Determination of Melting Point of Ultra-High Temperature Materials via Conical Nozzle Levitator
The Conical Nozzle Levitator (CNL) system has been used to measure the melting point of several high temperature materials. The CNL system uses lasers to heat aerodynamically levitated spherical samples (~3mm diameter) above their melting point. The temperature is measured in-situ via two single-color pyrometers. The samples are allowed to cool and freeze, and the observed thermal arrest upon freezing is determined to be the melting point temperature. Well characterized oxides (TiO2, Al2O3, ZrO2, HfO2) and oxygen sensitive refractory metals (Ti, Nb, Ta) were measured for calibration and uncertainty quantification.
Bio: Fox is a 5th year Ph.D. student in the Department of Chemical Engineering, advised by Professor Scott McCormack. His research is on the thermophysical properties of ultra-high temperature ceramics and materials. Fox's research has been published in the Journal of the American Ceramic Society, Journal of the European Ceramic Society, and Acta Materialia. He intends to graduate this summer.
FNU Meera, Materials Science and Engineering
Presenting: Structural response of silicate glasses at extreme conditions
Laser shock experiments give a unique insight into the behavior of the matter subjected to extremely high temperatures and pressures and are key to modeling material failure and deformation dynamics under ballistic impact. The dynamic response of silicate glasses is highly dependent on the glass network structure and composition. This fundamental understanding of the role of network structure, homogeneity, and atomic-scale mechanism for nanoscale plasticity will pave the way towards ‘compositionally tailored glasses’ that has the potential to achieve high intrinsic damage resistance under dynamic loading conditions critical for military and aerospace applications. Using in-situ X-ray diffraction (XRD) under laser driven shock compression we were directly able to resolve structural transformation in soda-lime (SLG) and borosilicate glasses (BSG) to clarify the role of composition on their shock response. We observed both amorphous to amorphous and amorphous to crystalline phase transitions influenced by the glass network structure. We present a novel pressure-density and pressure-time phase diagram for SLG and BSG from 40 to 300 GPa.
Bio: Meera is a sixth year Ph.D. student in the Department of Material Science and Engineering, advised by Professor Roopali Kukreja. Her research focuses on the understanding the behavior of Matter in Extreme Conditions.
Stefan Broecker, Computer Science
Presenting: ARDS Detection with Multimodal Machine Learning
Acute respiratory distress syndrome (ARDS), a severe form of hypoxemic respiratory failure with high in-hospital mortality, poses diagnostic challenges that can delay crucial interventions. Existing predictive models have made use of individual data sources that include ventilator waveform data (VWD), chest x-rays, and electronic health records to screen patients for ARDS, but no model has incorporated all three. This work uses a deep learning-based approach to combine the three modalities into a single predictive model to improve the accuracy and speed of ARDS detection. The CLIP-inspired approach could serve as a framework for use in other healthcare settings, where modalities with complementary information could be combined to improve upon the predictive performance of single-modality models.
Bio: Stefan Broecker is a third-year Ph.D. student in Computer Science advised by Dr. Thomas Strohmer. His research focuses on multimodal machine learning and synthetic data generation, in particular as it relates to data privacy. He is also interested in robustness and reliability in machine learning.