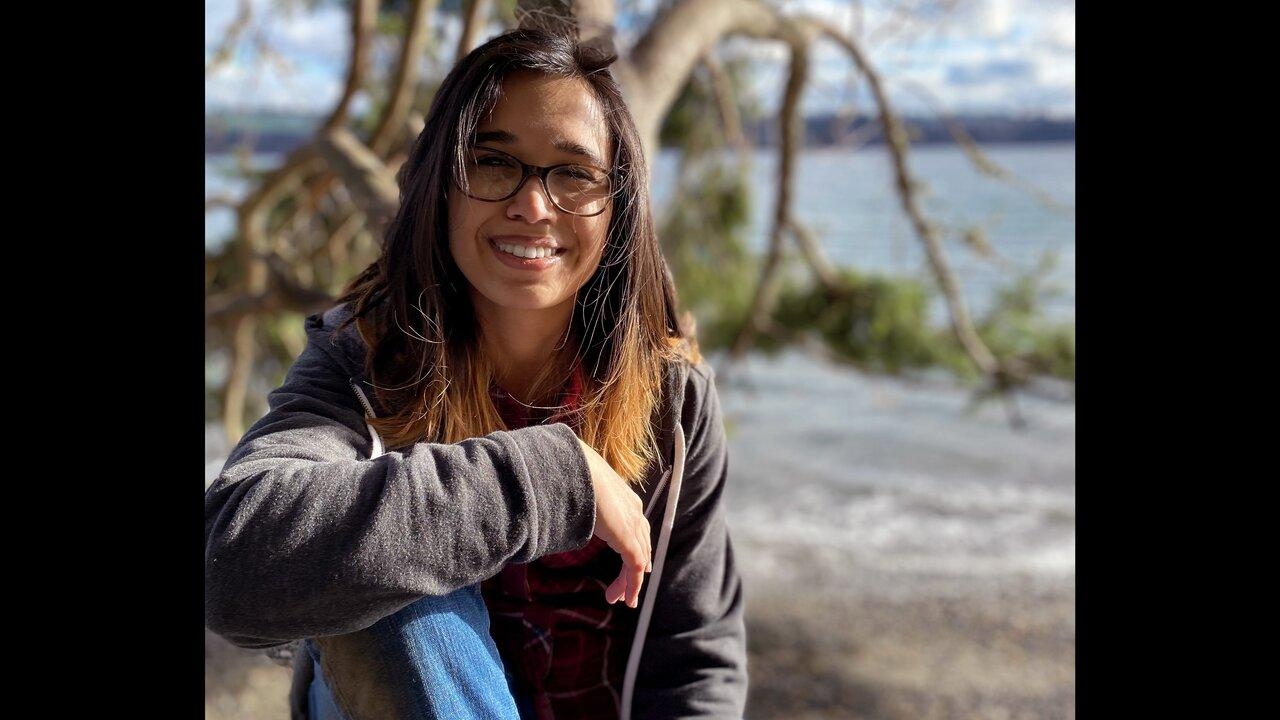
Meghna Srivastava Receives Jeffery C. Gibeling Award for Best Master’s Thesis
Meghna Srivastava is this year’s recipient of the College of Engineering’s Jeffery C. Gibeling Master’s Thesis Excellence Award. Srivastava earned her M.S. in materials science and engineering under the mentorship of Materials Science and Engineering (MSE) Associate Professor Marina Leite.
The annual award recognizes one M.S. student in the College of Engineering who has submitted the best master’s thesis. The award not only honors the individual student and publicizes their exemplary research, but also acknowledges the mentorship of their major professor.
The Gibeling Award is named in honor of MSE Professor Emeritus Jeffery Gibeling, who spent 14 years in leadership roles in UC Davis Graduate Studies, served as the college’s interim dean in 2021 and was the first chair of the Department of Materials Science and Engineering.
“It's an amazing honor,” said Srivastava. “Professor Gibeling is an excellent scientist and mentor, so I'm honored and humbled to receive a reward with his name attached. I'm also happy to know that folks are reading my thesis.”
An Automated Approach to Energy
In her thesis, “Automated Experimentation and Machine Learning for Perovskite Photovoltaics,” Srivastava outlines a data-centric approach to investigating the stability of perovskite photovoltaic materials.
Perovskites are extremely efficient in converting light into energy, which makes them perfect candidates for next-generation solar cells. However, they’re very prone to degradation in certain heat, light, humidity and electrical bias conditions, so finding perovskites that are stable in a wide range of environments is an important goal in the field. This can be done experimentally, but it would take years and millions of dollars to test every sample in every condition.
Srivastava laid out a road map for using data and machine learning as a solution. With the right data, machine learning algorithms can parse through these possibilities in a fraction of the time, pinpoint the most promising materials and accelerate product development.
To train her neural networks and statistical models, Srivastava developed a high-throughput automated experimental technique that uses environmental photoluminescence spectroscopy to acquire data on multiple samples in less than two seconds. Once training the model on enough of this data, she used it to accurately forecast the performance of perovskite materials under different conditions.
“My high-throughput characterization results and accurate time series forecasts illustrate the potential of data-centric approaches for perovskite stability investigations and showcase the promise of automation, data science, and machine learning as tools to drive photovoltaic solar cell commercialization,” she concluded in her abstract.
“Meghna’s M.S. project is at forefront of materials research, encompassing renewable energy materials and machine learning,” said Leite. “She did a great job of developing the instrumentation needed for high-throughput optical measurements of perovskite solar cells and by quantitatively comparing distinct machine learning methods when predicting materials' degradation.”
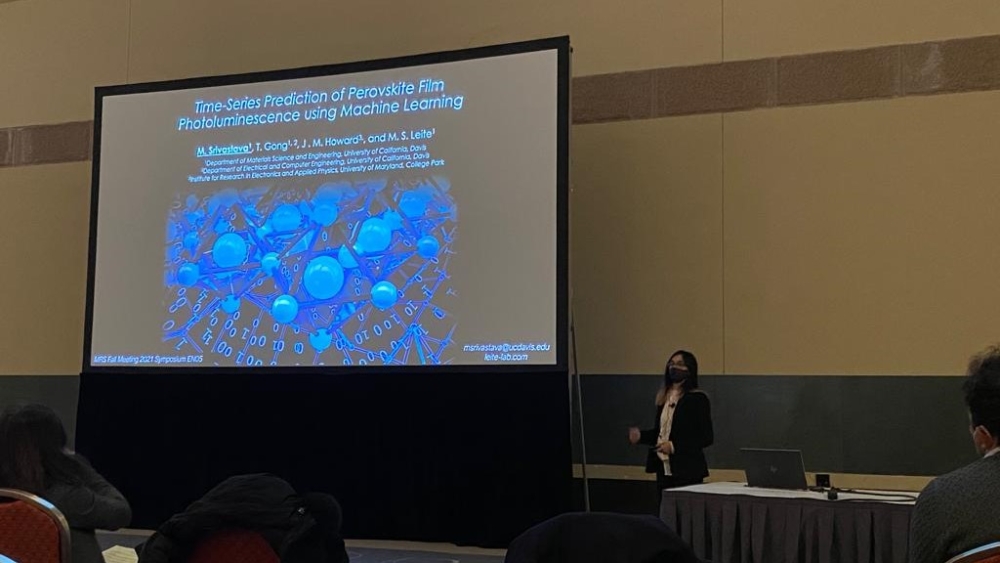
Award-Winning Student
As a M.S. student, Srivastava received several awards and honors, including two best paper awards and a National Science Foundation Graduate Research Fellowship in 2020. Her abstract was also one of only 10% selected to be presented in a symposium at the fall 2021 Materials Research Society (MRS) conference.
Srivastava graduated with a B.S. in materials science and engineering from Cornell University and joined UC Davis in 2019. After meeting Leite, she became interested in the idea of using machine learning for energy applications and decided to join the lab for her graduate work.
She finished her M.S. in March 2022 and now works as a data engineer for Voltus, an energy technology company that manages energy load during demand peaks. She says she uses a lot of the data analytics and coding skills she developed at UC Davis to make a difference in preventing blackouts and increasing the energy efficiency of the grid.