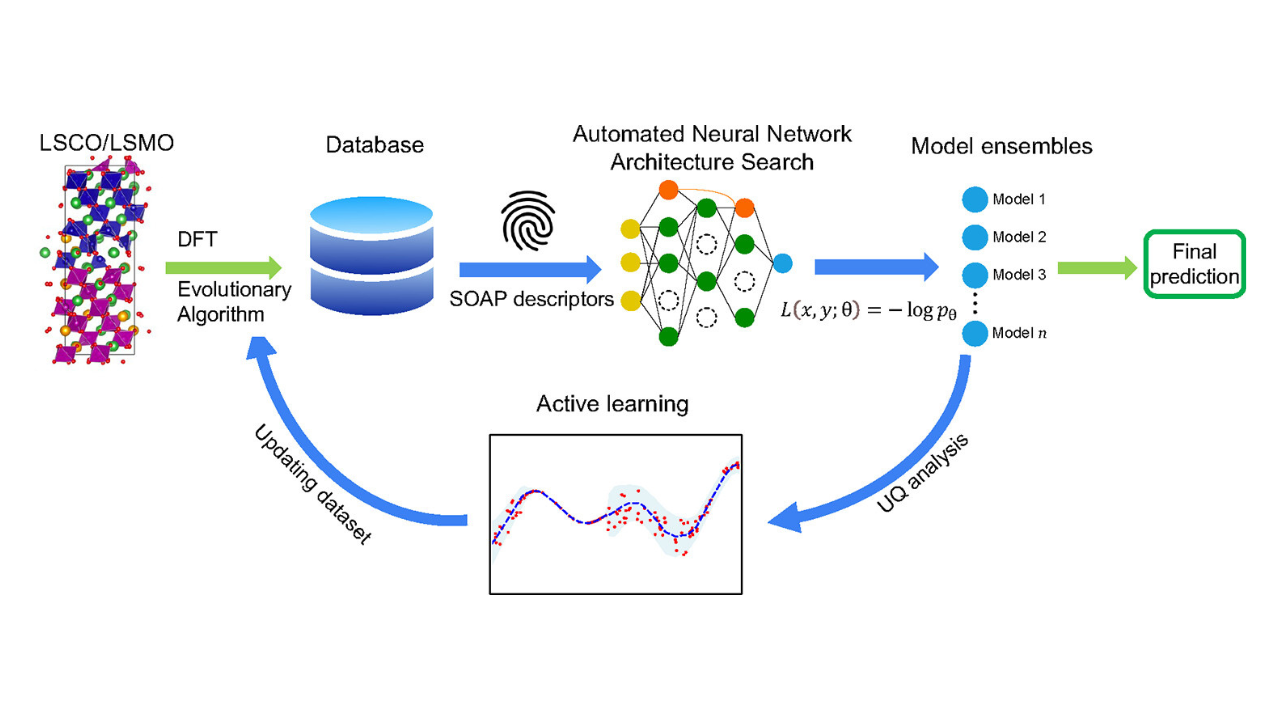
Partnership with National Lab Leverages New Computational Techniques
Yayoi Takamura, professor and chair of materials science and engineering at the University of California, Davis, and researchers at Lawrence Livermore National Laboratory, or LLNL, have designed deep-learning model ensembles, a method in machine learning involving multiple neural networks, to investigate the magnetic behavior of perovskite oxide multilayers.
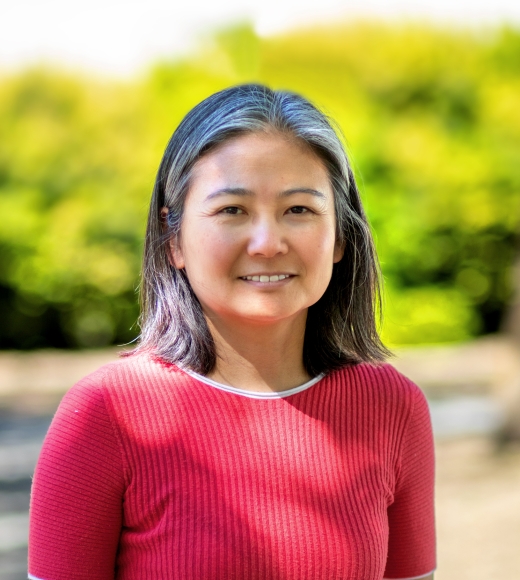
Perovskite oxides are gaining attention for use in next-generation magnetic and ferroelectric devices due to their exceptional charge transport properties and the opportunity to tune the different properties of electrons and atoms, including charge, spin, lattice and orbital degrees of freedom.
While the materials may offer a pathway for innovative designs in perovskite oxide-based devices, the atomic-level compositions of the interfaces between perovskite oxides are unknown, therefore hindering the establishment of design principles using these materials.
With this new model, the researchers investigated the effects of composition and process parameters on the magnetic behavior of perovskite oxide multilayers.
For the model, the researchers leveraged first-principles simulations using density functional theory, or DFT, a quantum mechanical method that uses electron density to calculate electronic structures of many-body systems like solids, liquids and gases; evolutionary algorithms; and neural network searches with on-the-fly uncertainty quantification, which estimates uncertainty during calculations and can enhance accuracy.
This approach allowed the researchers to screen over 50,000 interface structures for the most stable ones, while accurately describing their magnetic properties. The research, published in ACS Applied Materials & Interfaces, provides valuable insights into designing perovskite oxide multilayers, enabling tailoring of their functional properties to meet specific requirements for various device applications and opening new avenues for more efficient screening of materials with multiple components.
"This partnership with LLNL allowed us to harness their computational expertise to develop the workflow needed to understand emerging phenomena at perovskite oxide interfaces,” said Takamura. “The newly developed workflow allows us to quickly and efficiently explore the full parameter space and can be applied further to other materials problems."