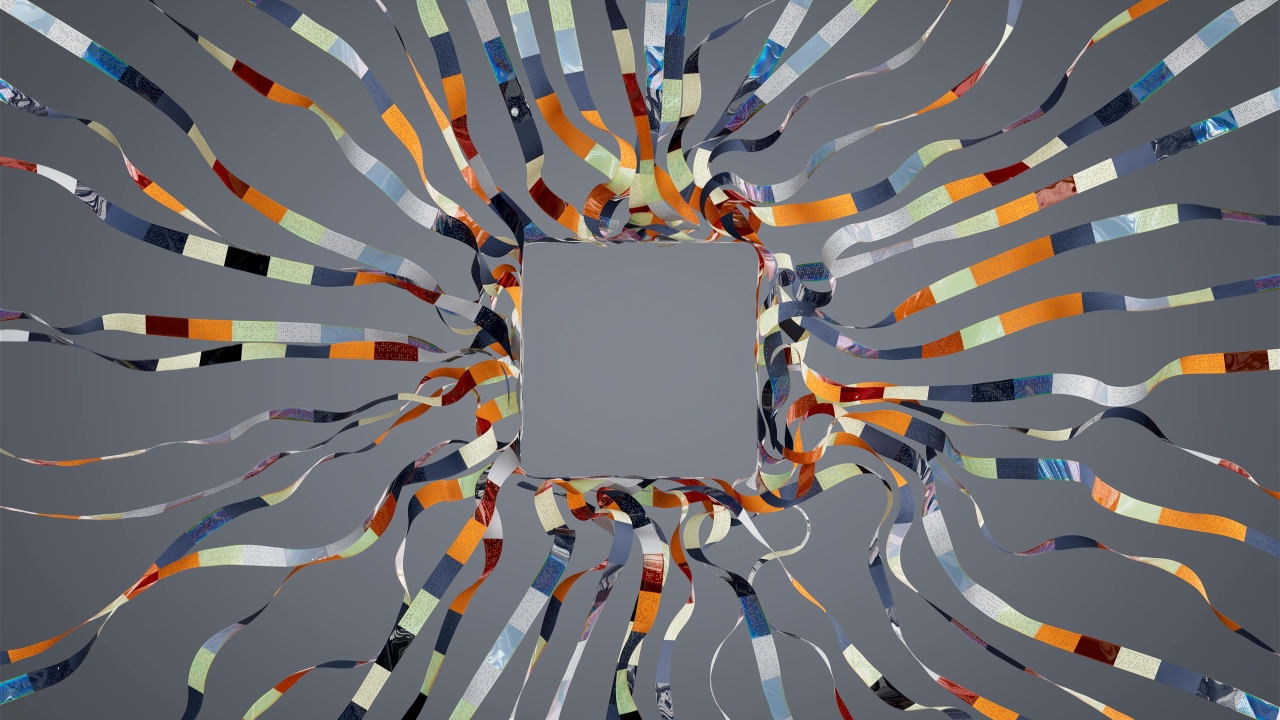
New Model May Change Artificial Intelligence for Good
Electrical and computer engineering professor leads innovative project that automates the design of neural networks
Artificial intelligence designing other AI? It's a futuristic reality we're closer to than ever before.
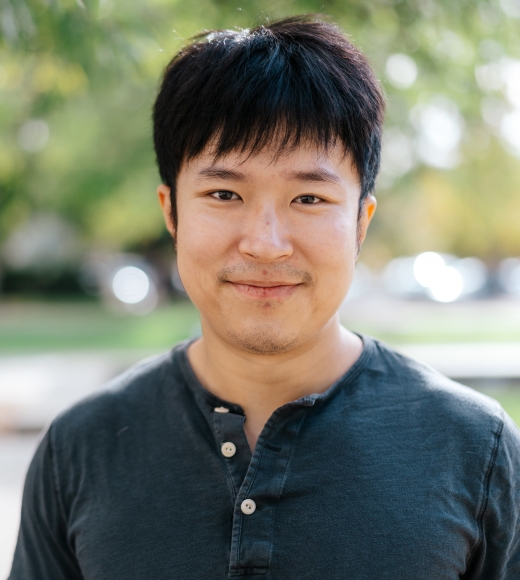
AI models can now create and test new models with minimal human intervention thanks to a collaborative effort spearheaded by Silicon Valley-based artificial intelligence startup Aizip and researchers at the University of California, Davis. Researchers at the Massachusetts Institute of Technology, UC Berkeley and UC San Diego also contributed to the project.
The innovative technology uses multiple AI models that work together to find, generate and label data, removing the need for researchers to select and annotate millions of samples to train new models. The technology can also build AI in specific architectures from the ground up, such as a recurrent neural network or a convolutional neural network.
"For the first time, we can design an AI model from data preparation to model deployment automatically with AI design automation," said Yubei Chen, an assistant professor of electrical and computer engineering at UC Davis and chief technology officer for Aizip who led the collaborative project.
An immediate benefit of this technology is the reduced manual labor in creating neural networks, which could significantly reduce production costs and make the technology more accessible.
"The goal is not to remove the human element but to make the process less labor-intensive and more elegant," Chen said.
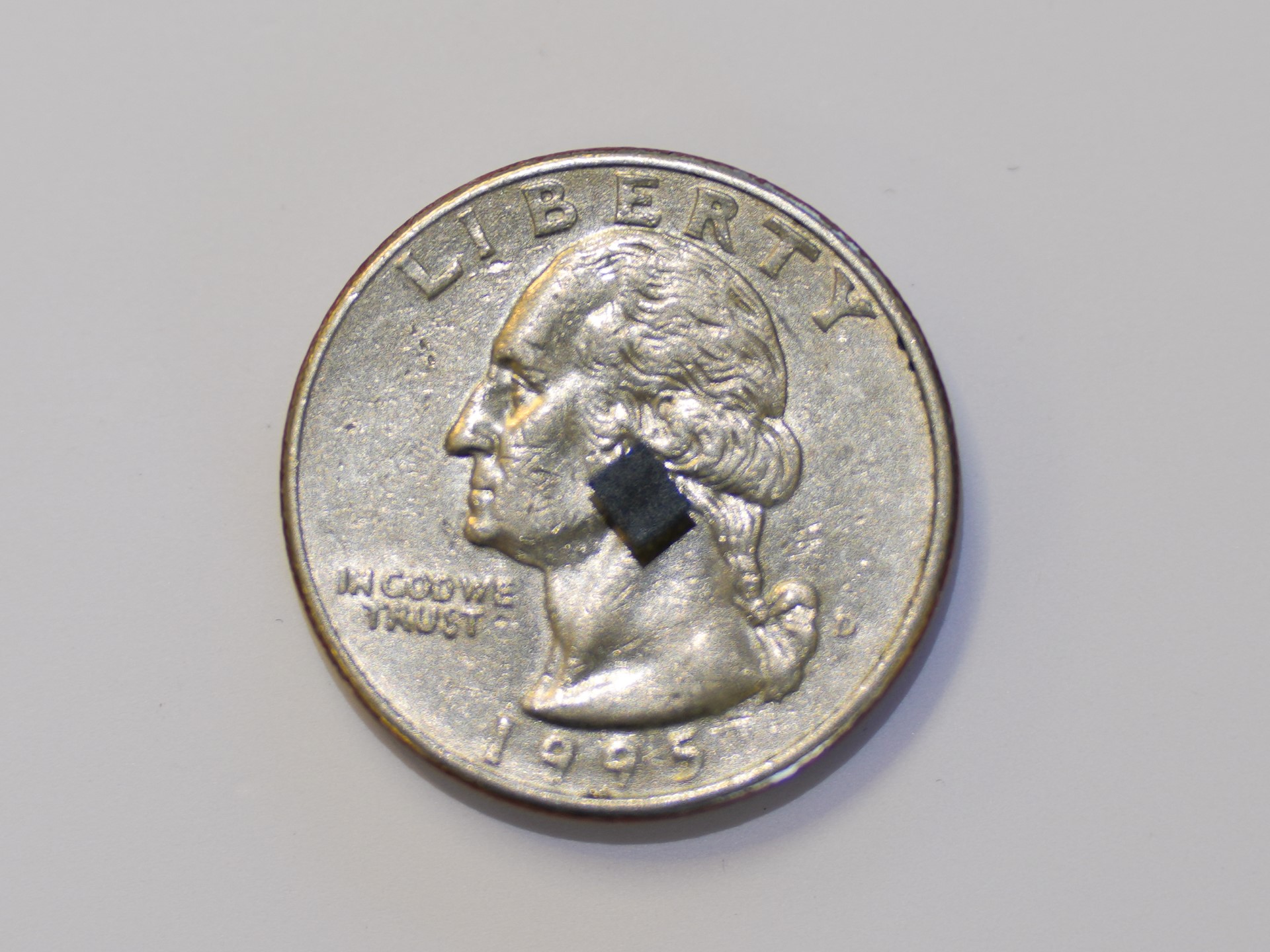
The automated design paradigm also promises to power the future of pervasive AI, or where everyday objects, from sneakers to sprinkler systems, have the ability to think and adapt over time.
In terms of research, the new model may signal a shift in how engineers think about researching efficient AI. Often, efficiency is considered through the lens of writing faster code, Chen explained. He wants to move the conversation toward better data.
Researchers typically train AI by feeding it thousands upon thousands of data samples. These samples form the knowledge base of the model and inform its decision making. Chen's approach is about being more intentional with the data used to train neural networks.
To elaborate on this idea, he gave the example of helping someone make spaghetti for the first time. Rather than showing that person an entire cookbook, it would be better to provide them with the page about making spaghetti because they would immediately have the precise information needed to make the dish. There would be no need to compare recipes for more information or to spend time locating the exact page.
The models at the core of this new technology feature such a lean, data-centric design approach. The thought is that with more curated data, the more efficient the AI models become, without the need for more efficient code.
Chen hopes this data-centric methodology continues to develop in academic conversations, as he believes it is key to taking AI to the next level over the next few decades.
"We're at the forefront of transforming efficient AI design," Chen said, reflecting on this research project, his efforts as a UC Davis researcher and the AI research community in general. "Our commitment is not just to speed up and refine the process but also to tackle the fundamental questions in efficient and pervasive AI."