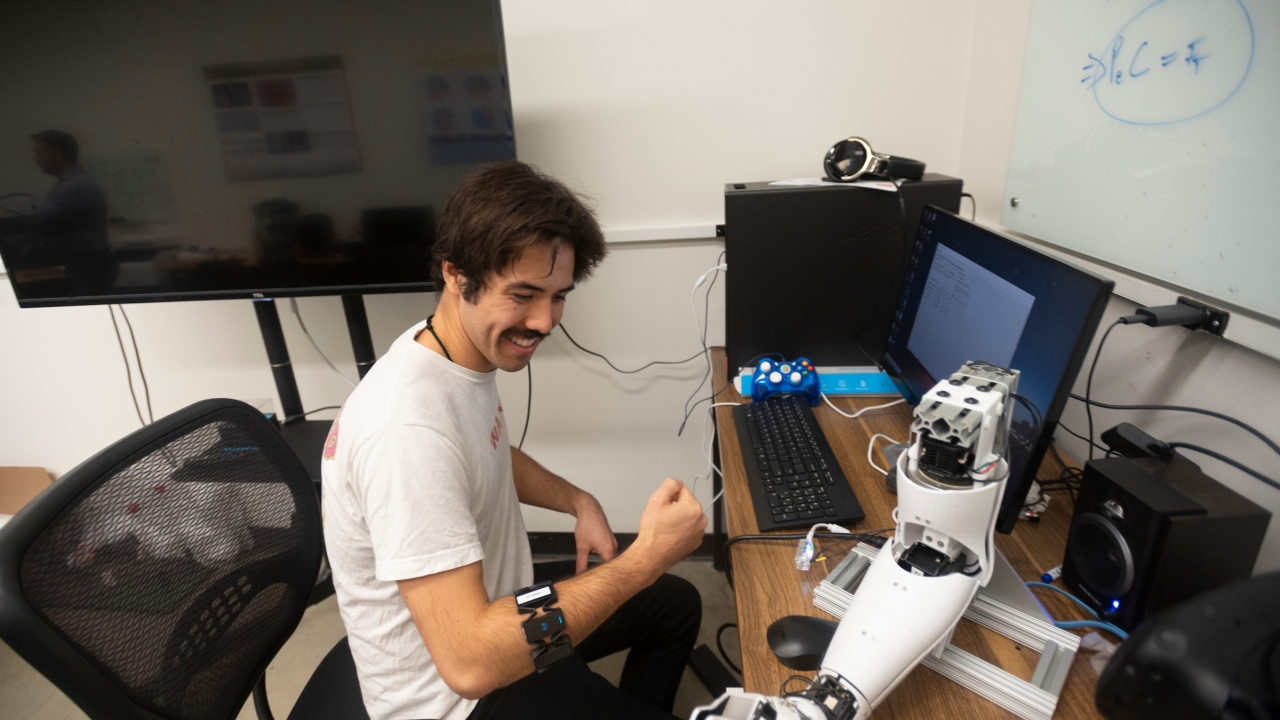
Combining Signals Could Make for Better Control of Prosthetics
Combining two different kinds of signals could help engineers build prosthetic limbs that better reproduce natural movements, according to a new study from the University of California, Davis. The work, published April 10 in PLOS One, shows that a combination of electromyography and force myography is more accurate at predicting hand movements than either method by itself.
Hand gestures such as gripping, pinching and grasping are driven by movements of muscles in the forearm. These movements generate small electrical signals that can be read by sensors on the skin, a technique called electromyography, or EMG.
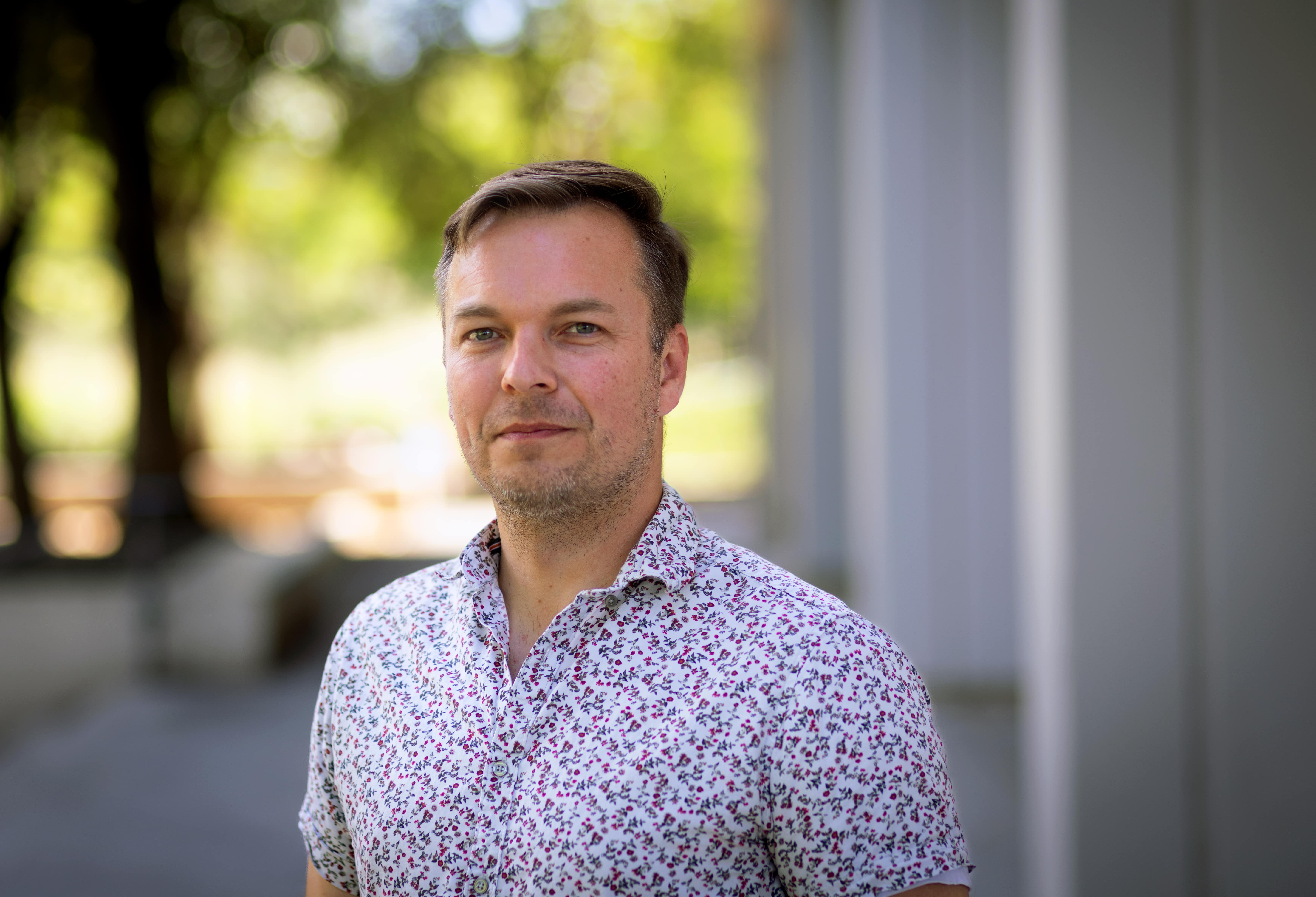
“Using sensors and machine learning, we can recognize gestures based on muscle activity,” said Jonathon Schofield, assistant professor of mechanical and aerospace engineering at UC Davis and senior author on the paper.
EMG-based controls perform well in a lab setting and with limbs at rest. But there is a well-known problem of “position and load.” If you move your arm to a different position — say, shoulder height, or over your head — or grasp objects of different weights, the measurements change.
“In the real world, every time you move a limb and grasp something, the measurement is going to change,” said Peyton Young, first author on the paper and a Ph.D. student in mechanical and aerospace engineering. “The neutral position (where the limb is held passively next to the body) is very different to moving around.”
Combining EMG and FMG
To address this, Young and Schofield experimented with a different type of measurement, alone and in combination with EMG. Force myography, or FMG, measures how muscles in the arm bulge as they contract.
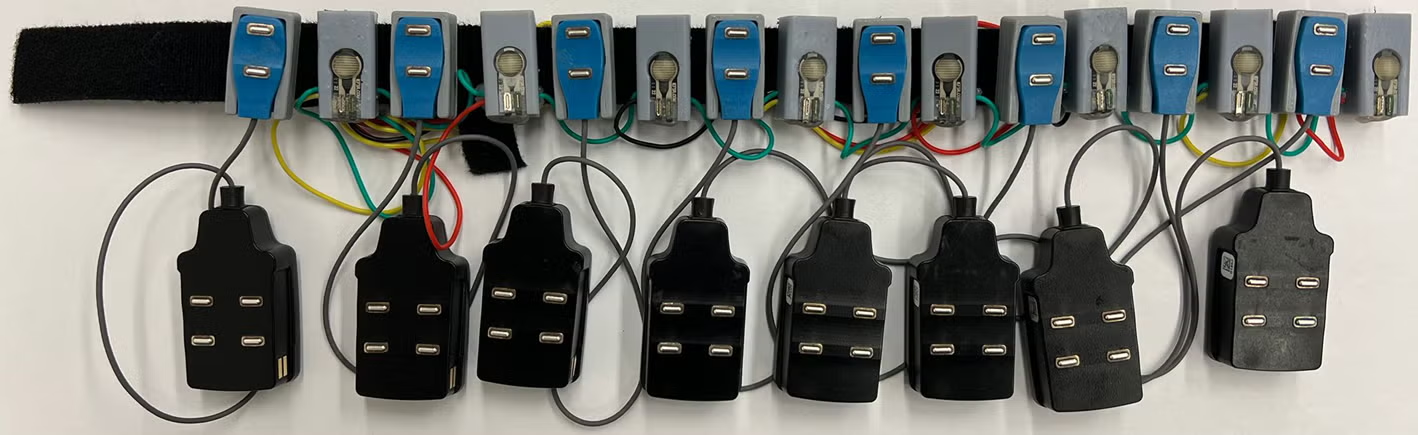
Young constructed a cuff that goes around the forearm and includes both EMG and FMG sensors. He used this device with a series of able-bodied volunteers in the lab who performed a series of arm gestures while participants held different loads with different hand grasps.
Data from the sensors was fed to a machine learning algorithm to classify the different movements into pinch, pick, fist and so on. The algorithm was trained on either EMG or FMG signals alone, or on a combination.
For each experiment, the algorithm was trained on some of the data and scored on its ability to accurately classify the rest.
“We train the classifier on data from the gestures, then score it on its ability to predict them,” Young said.
They found that position and loading did indeed affect the accuracy of the classification of gestures. Overall, a combination of EMG and FMG gave over 97 percent classification accuracy, compared to 92 percent for FMG alone and 83 percent for EMG alone.
Young is now working on a combined FMG/EMG sensor, and the team is working towards an experimental prosthetic limb that uses the technology.
The approach could have a wide range of applications for prosthetics and robotics as well as for virtual reality tools, Schofield said. The team benefits enormously from being able to collaborate with clinical prosthetics experts, surgeons and biologists across UC Davis, he said.
“We wouldn’t be able to do it without exposure to actual patients and clinicians,” Schofield said.