Beneath the Surface
Maike Sonnewald Decodes the Ocean with AI to Build a More Resilient Future
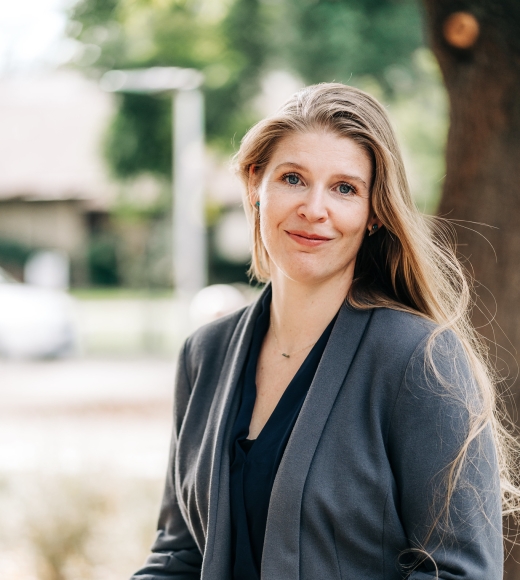
(Steven Trinh/UC Davis)
At the AI for Good Global Summit hosted by the United Nations’ International Telecommunication Union in May 2023, Maike Sonnewald, assistant professor of computer science, told her audience that one of the first big computers ever made was for weather prediction and chaos theory.
With artificial intelligence and machine learning as the next evolution in modeling and data mining, Sonnewald believes AI has the potential to be at the core of keeping people safe from extreme weather events. Sonnewald is using neural networks and other machine learning tools to deepen our understanding of the ocean to better predict long-range weather. Being able to predict the future of weather can strengthen our resilience to climate change by preparing us for what’s coming as weather becomes increasingly erratic.
We spoke with the oceanographer-slash-computer-scientist about her pioneering use of machine learning to plunge into the unknown depths of the ocean and bring insight into the ocean’s role in humanity’s future on the Earth’s surface.
How did you become interested in studying the ocean through computer science?
The ocean is one of the most important drivers of Earth’s climate and one of the hardest things to observe. We can observe the atmosphere and we can observe the land fairly easily, but to get data from the ocean, you have to go there with either your autonomous robot or ship, and that's very expensive and hazardous. The questions that pertained to how we can learn more, how we can run models faster and bigger, and how we can use things like machine learning to learn more about the ocean — the usefulness of data mining and sparse data inference, for instance — was very obvious to me. So, I decided to go into oceanography and computer science.
The ocean can be an extremely unforgiving environment to gather data from, as you mentioned. How are you using AI to combat this challenge?
What I have tried to do and what I've successfully done for climate models is take data from the surface and effectively infer subsurface circulation. That's one area where the computer science expertise that I have really comes in handy to be able to guide what we can do, what is possible, what the frontiers are and how we can move those forward in a way that targets and develops these AI methods toward what we need.
Right now, we’re developing a neural network methodology — a model made up of interconnected nodes that are organized into layers and work together to propagate information and identify patterns to ultimately make predictions and decisions similar to the way a human brain would — that is able to be verified, so I can actually confirm that this “black box” is looking at something using similar reasoning as one does with conventional physical fluid dynamics.
How can using AI to produce ocean models make climate predictions better?
First, we can use AI to speed up the traditional models by being able to point out what's missing. We can ask a climate model if it's got the right processes in it for making its predictions. For example, if a current is moving away from the coast, why is it doing that? Are the right processes interacting, for instance, is the wind pushing on the surface being balanced by rubbing against the bottom? If not, why? This approach can speed up the development of the model, whereas one would otherwise have to comb through huge amounts of data and run the model many times before it becomes clear.
Second, we can build entirely new forecasting models with AI using parts of existing models that are much too computationally expensive to use otherwise. This is called an emulator because it's effectively an AI copy of an existing model. With these, we can run simulations more efficiently than traditional models because the AI models are orders of magnitude faster. These methods are still under development in many areas, and my group is interested in how we can include the ocean in a better way for local predictions of long-range weather.
Third, we can use AI to fill in the gaps of models that are low-resolution, or very pixelated if we're using a photograph analogy. Low-resolution models are easier to run for simulations over hundreds of years, but the things they can't "see" can be problematic and cause uncertainty in the predictions. AI is being explored as a way to fill in this gap using data from models that have much higher resolution.
Where have you seen breakthroughs in your research so far?
My research into the Southern Ocean super gyre contributes to resilience by providing fundamental knowledge of how the southern branch of the overturning circulation is working, and thus, our ability to know where to sample from and how likely it is to drastically change.
Just modeling the Southern Ocean is hard because it has a unique geography: it doesn't have any continents blocking the currents. There's a really big, very important current that sort of whizzes around because it's not stopped by anything called the Antarctic Circumpolar Current, or ACC.
I was able to use unsupervised learning, or data mining, of data to elucidate the fact that previously we've looked at certain circulations, like the ACC, without the full picture. I highlighted that those areas not normally observed — because they are under ice or even harder to get to — are important to consider as they are part of one big system.
What are the challenges and benefits of using AI in oceanography research?
Some of the main challenges with AI tools are that they are not always ‘fit for purpose’ and that we’ve not quite figured out how to use them yet. It’s a bit like trying to use a hammer to hit a screw: It works but is not the best tool for the job.
Using AI, we can not only do ‘old’ things like numerical modeling faster or more accurately — which is hugely important for policy decisions — but also entirely new things, and thus accelerate progress in understanding. I would also stress that we can use AI to be more objective. It is hard as humans to not be guided by what we think we should see and look for patterns that we think should be there, a bit like when we ignored that there was an ozone hole because we didn’t think it should be there, but of course, it was, and we needed to take action.
To really accelerate progress and understand the ocean’s role in the climate system, we need more fundamental knowledge and insight.
Learn about how we are strengthening climate resilience