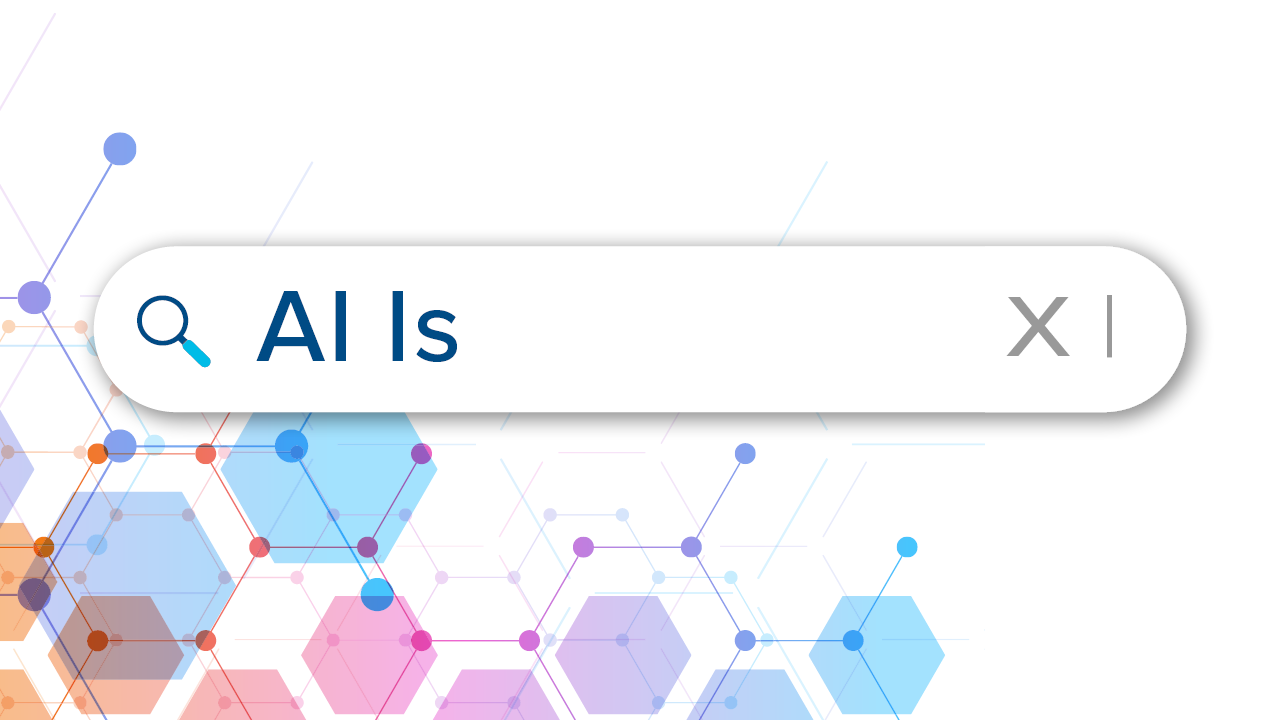
AI Is
Technologies like ChatGPT have brought the potential of artificial intelligence, from writing emails to optimizing a website’s code, into public awareness unlike ever before.
The thing is, the applications and implications of AI go far beyond large language models like ChatGPT. AI has the potential to detect cancer, build climate resilience and reveal humanity at its best and worst. It is the promise of a new era where humans can achieve the previously unthinkable.
With a new center for AI on the horizon and research projects using AI to advance healthcare and food systems, to name a few, the UC Davis College of Engineering is quickly becoming a hub of ingenuity and research dedicated to making AI safe, accessible, trustworthy and foundational. Welcome to the new now, and welcome to all that AI is and can be.
AI Is a New Frontier
By harnessing the power of neural networks and studying the natural mechanisms of the human brain, professors in the Department of Electrical and Computer Engineering are exploring the furthest limits of artificial intelligence.
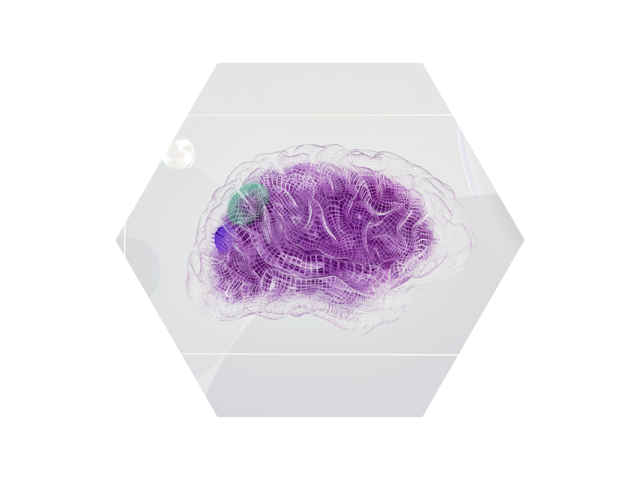
Mimicking the Human Brain
Current computers, which rely on information encoded in ones and zeroes, require high rates of power consumption to keep up with algorithms and machine-learning tools. To address this issue, Distinguished Professor S. J. Ben Yoo is leading a five-year, $4.75 million project to develop a first-of-its-kind computer architecture that communicates in electrical impulses like the human brain.
The human brain is incredibly efficient at creating and processing information, needing only 20 watts of energy to develop complex thoughts and solutions to difficult problems (about what it takes to power a lightbulb). In addition, the brain-like architecture may make it easier for artificial intelligence models to deal with unpredictable information and events, much like humans can and current AI models can’t.
Refining Neural Network Design
Assistant Professor Yubei Chen’s data-centric approach is signaling a shift in how engineers think about efficient AI.
Researchers typically train AI by feeding it thousands upon thousands of data samples to form the knowledge base of the model and inform its decision-making capabilities. While the classical approaches in efficient AI are mostly about models or systems, Chen's approach is about being more intentional with the data used to train neural networks.
Chen has already applied this lean approach to create an AI model that can build and design smaller AI models with minimal human intervention. Ultimately, Chen’s research is in pursuit of a world model in AI systems capable of imagining or dreaming scenarios. Such a world model can serve as a scalable data simulator, which will power data-centric efficient AI development.
AI is Changing Food Systems
Climate change and a growing global population are creating an urgent need to improve food systems. These researchers are using AI to build efficient, sustainable agriculture.
Embracing Potential
Mason Earles, an assistant professor in the Departments of Biological and Agricultural Engineering and Viticulture and Enology, testified to the U.S. Senate Committee on Agriculture, Nutrition, and Forestry on the potential of AI to grow better food more efficiently.
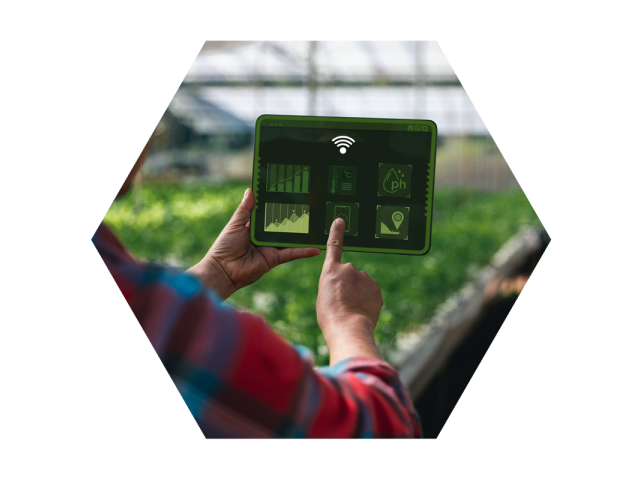
He voiced the need for pathways for computer science students to enter the agricultural and food sectors to foster innovation and collaboration in using AI to solve challenges in the field.
AI centers like the AI Institute for Next Generation Food Systems, or AIFS, which is led by computer science professor Ilias Tagkopoulos and for which Earles is co-principal investigator and agricultural production lead, need continued and expanded funding, Earles argued, to tackle the growing demands of agriculture and secure the United States as a global leader in agricultural innovation.
Mechanizing Agriculture
Stavros Vougioukas, professor and vice chair of the Department of Biological and Agricultural Engineering and researcher for AIFS, works to advance robotics and mechanization in agriculture.
In 2021, the National Science Foundation and the National Institute of Food and Agriculture provided $1.6 million to support his development of two fruit-picking machine concepts. One concept combines cameras and neural networks to identify harvestable produce and uses optimally coordinated robot arms to pick them, whereas the other uses shake-and-catch mass-harvesting. He also helped develop fragile crop harvest-aiding mobile robots, which safely transport damage-prone crops, potentially helping improve wages and reduce cost and production risk.
AI is Biased but Teachable
Is AI good or bad? When asked, ChatGPT said, “Artificial Intelligence is inherently neutral; it doesn't possess inherent morality.” However, research shows AI does have a bias problem. In an effort to understand how AI learns, these researchers are getting to the core of AI’s decision-making.
Fair and Explainable
Ian Davidson, a professor of computer science, is researching bias in AI programs and how to make them as fair as possible as well as explainable, meaning the AI can explain why it made the decision.
In one experiment, Davidson asked an algorithm to sort faces into unusual-looking people and normal-looking people. The AI determined that “normal-looking” was largely blond, white and female, and “unusual-looking” was largely Black, Asian and male.
Now he is exploring how to code fairness into AI algorithms.
In another project, Davidson worked with UC Davis Health physicians to teach a functional MRI scanner to help determine treatment for young adults with schizophrenia by comparing their scans against previous patients’ scans. The machine performed with over 80% accuracy, so now the challenge is having the machine explain how it came up with the results.
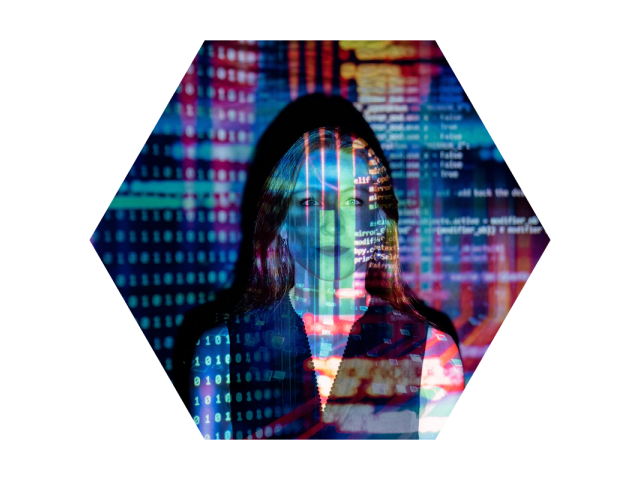
Down the Rabbit Hole
Associate Professor of Computer Science Zubair Shafiq and Ph.D. student Muhammad Haroon are on a multidisciplinary research team that audited YouTube's video recommendations in 2021 and 2022.
The study included watching nearly 10 million YouTube videos from multiple “sock puppet” accounts, or automated accounts that mimic a person, using the platform with right- and left-leaning political preferences, to reveal how the algorithm treated each fake, curated profile.
Not only did researchers find that the political content was biased towards the right- and left-leaning sides, but the level of extremism in the content was also disproportionately distributed to the right-leaning profiles, with more frequent recommendations for videos containing conspiracy theories and polarizing content.
AI is Advancing Healthcare
Artificial intelligence can be an extremely valuable tool in the healthcare field, with biomedical engineering and electrical and computer engineering researchers using it to create next-generation medical advancements that promise to transform patient care.
Bringing Medical Imaging to the Next Level
Simulations of how light particles interact in nuclear imaging devices, like CT and PET scanners, are crucial for optimizing the scanners’ ability to produce high-quality images of the human body, potentially making it easier to detect abnormal structures in the body, like cancer.
Associate Professor of Biomedical Engineering Emilie Roncali is working to improve the efficiency of these images by designing new detectors through computer simulations powered by generative adversarial AI. Called optiGAN, the neural network will cut the time needed to simulate and track the light particles, offering critical insights for developing ultrafast detectors and ultimately more detailed medical scans.
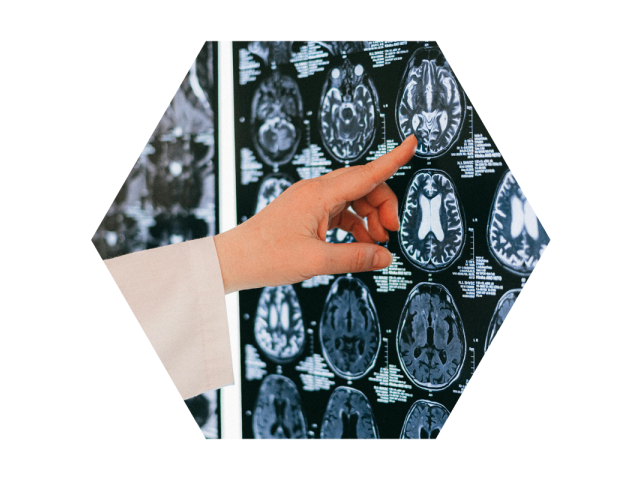
Detecting Cancer
Electrical and computer engineering professor J. Sebastian Gomez-Diaz and biomedical engineering associate professor Randy Carney are working together to develop a novel nanotechnology that is a condensed version of a platform for infrared absorption, or IR, spectroscopy. IR spectroscopy is a highly accurate analytical instrument that can be used for clinical diagnosis of cancer but typically involves large, cumbersome machines, complex data and high costs to implement.
Gomez-Diaz and Carney are pairing the device with a neural network that will learn to detect the specific signs of cancer within an individual’s biospecimen sample, like blood or saliva. The team hopes that this combination of miniaturized hardware and artificial intelligence will simplify and shorten the diagnostic process while providing a much more accessible and less invasive way of detecting cancer.
Accessible Health
Professor of Electrical and Computer Engineering Chen-Nee Chuah uses data science and machine learning techniques to improve on- and off-site patient care with smart devices. She’s creating an AI-assisted, real-time clinical decision support system that works alongside doctors for labor-intensive tasks, such as around-the-clock monitoring of patients in the intensive care unit and mechanical ventilator management for early detection of acute respiratory lung syndrome.
She has co-led several interdisciplinary research projects with colleagues from UC Davis' School of Medicine and School of Nursing to improve critical care and maternity care, especially for underserved populations. Chuah is also developing computational, data- and label-efficient deep learning frameworks for neuropathology image analysis tasks to aid deeper phenotyping of neurogenerative and cerebrovascular diseases, such as Alzheimer’s disease and stroke.